Health Informatics: As the amount of biological data grows, data scientists can help analyze and interpret large amounts of this information.
Master’s in Data Science—Your Guide to Data Science Graduate Programs in 2023
Our site offers prospective and current data science students information on different degree options, bootcamps, short course offerings, career choices post-graduation, and resources for staying current in the field once they begin to practice. Even professionals in the middle of a career transition can benefit from our research-based articles, which include job growth trends and up-to-date salary data for an array of positions in data science.
Sponsored Online Master’s in Data Science Programs

The University of North Carolina at Chapel Hill
School of Information Studies
Master of Applied Data Science
UNC-Chapel Hill’s online Master of Applied Data Science program gives you a holistic understanding of the data life cycle, preparing you to effectively — and ethically — collect, process, manage and analyze data. Learn to translate your insights into a clear narrative that can be used to drive action. Math and programming skills required.
- Complete in as few as 16 months full time.
- No GRE scores required.
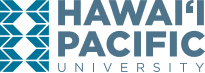
Hawaii Pacific University
College of Natural and Health Sciences
Master of Science in Data Science
The online MS in Data Science program from Hawai‘i Pacific University prepares students to interpret both conventional and unconventional data, strengthen creative problem-solving skills, and apply cutting-edge data analysis expertise to nearly every industry.
- 12 months to complete full time and 24 months part time
- Standardized test scores not required (No GRE/GMAT)
- Students learn key skills such as data analysis, data mining, data visualization, programming, machine learning, and more

Southern Methodist University
Dedman College of Humanities and Sciences, Lyle School of Engineering and Meadows School of the Arts
Master of Science in Data Science
Earn your MS in Data Science at SMU, where you can specialize in Machine Learning or Business Analytics, and complete in as few as 20 months.
- No GRE required.
- Complete in as little as 20 months.
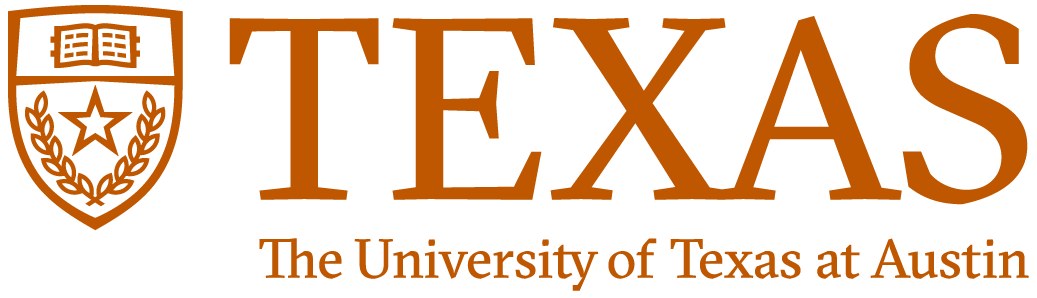
University of Texas at Austin
Dedman College of Humanities and Sciences, Lyle School of Engineering and Meadows School of the Arts
Master of Data Science
In today’s data-driven world, there’s an increased need for data scientists in every industry. Accelerate your career in this rapidly growing field by completing a rigorous master’s degree program that’s 100% online, affordable, and flexible to fit your life. With an MSDS from the top-ranked University of Texas at Austin, you’ll gain in-demand skills in data visualization, data mining, data analysis, machine learning, and more.
- Top Ranked University
- Complete in 1.5-3 years
- Foundational data science curriculum
SPONSORED
WHAT IS DATA SCIENCE?
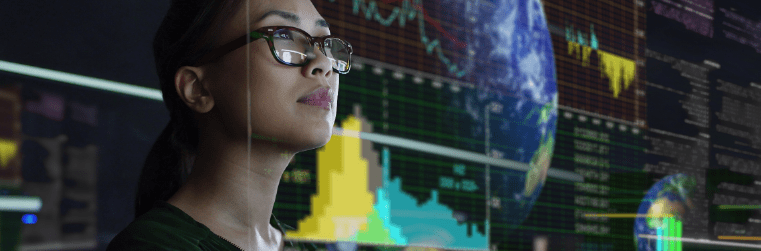
Data science is a dynamic field that’s becoming increasingly valuable to many companies, small, large and mid-size. From capturing data to communicating results, data scientists play an important role in helping businesses make strategic decisions and optimize outcomes. Where it traditionally encompassed data mining, programming skills, and analyzing sets of data, data science today is expansive and involves looking at the entire data science life cycle.
“Hiding within those mounds of data is knowledge that could change the life of a patient or change the world.”
— Atul Butte, M.D., Ph.D., University of California, San Francisco
In the business world, data science is used to help corporations develop an understanding of consumer and market trends. In other arenas, data science is used to collect information, but usually has different end goals such as gathering census data to be used by public policy professionals or statistics about diseases to inform public health policy, recommendations and medical solutions.
DATA SCIENCE PROGRAMS AND RELATED DEGREES
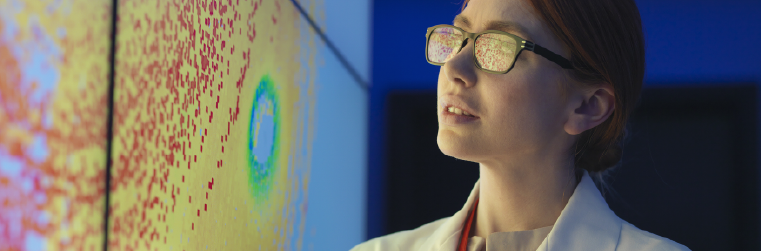
From machine learning and artificial intelligence to data systems administration and security, there are a variety of focus areas and types of programs you can pursue through higher education. Some of your options include master’s degrees in data science, bachelor’s degrees in data science, bootcamps, and short courses.
Master’s in Data Science
Earning a master’s in data science can help you gain a broad skill set that can be applied to a vast number of tech-related careers, such as data engineering, data architecture, or computer programming.
As a student in this advanced degree program, you can expect to dive into essential concepts in the following areas:
- Applied Statistics
- Database Systems and Data Preparation
- Practical Machine Learning
You will also learn how to use programming languages like Python, R, and SQL.
Master’s in Business Analytics
Business analytics uses data science to understand and predict consumer, market, and world economic trends. Earning a master’s in business analytics can help you develop the skills needed to transform large amounts of data into actionable business strategy insights.
As a student in this advanced degree program, you will gain expertise in these subject areas:
- Programming
- Data Analytics and Management
- Data Models and Visualization
For business analytics enthusiasts, “data-driven decisions” is the name of the game.
Master’s in Information Systems
To architect new technologies for companies, you’ll need to be well versed in using information systems and operations. Earning a master’s degree in information systems can help you develop the skills needed to build and manage information systems, evaluate technical approaches and risks, and communicate and manage people in organizations.
As a student in this advanced degree program, you will gain expertise in these subject areas:
- Information Systems Analysis and Design
- Business Telecommunications
- Managing Emerging Information Technology
Other Related Master’s Degrees in Data Science
Earning a master’s degree in a related discipline such as computer science, computer engineering or cybersecurity can prepare you for a career in data science, and is becoming increasingly valuable. The U.S. Bureau of Labor Statistics reports a 21 percent growth in jobs for computer science and 35 percent growth for information security jobs from 2021 to 2031. The projected growth for both occupations exceeds the 5 percent growth rate for all occupations during the same time period.
Computer Science Careers
- Computer Scientist
- Assistant/Adjunct Professor of Computer Science
- Computer Systems Analyst
- IT Project Manager
- Director of Web Development
- Chief Technology/Information Officer
Learn more about an online master’s in computer science to jump-start your career.
Computer Engineering Careers
- Mechanical Engineer
- Electrical Engineer
- Computer Network Architect
- Research and Development in Physical, Engineering and Life Sciences
Explore starting your career in computer engineering with a master’s online.
Cybersecurity Careers
- Digital Forensics Analyst
- Information Security Project Manager
- Systems Security Administrator
- IT Security Consultant
- Chief Information Security Officer
Discover online Master’s in Cybersecurity programs to kick off your career.
There are also hybrid programs for those looking to earn a master’s in data science in a specific industry—like earth sciences or health care—that offer more specialized experience.
Geological Information Systems (GIS): For the field of geology, GIS are a vital tool in unearthing geographic data, analyzing spatial information, and even in editing data in mapping.
Data Analysis for Public Policy: The field of public policy makes heavy use of data in order to interpret domestic and world trends in fields like population, mortality, and education levels.
Bootcamps
Bootcamps provide a unique opportunity for individuals to reskill or upskill their current technical knowledge in areas like data science, analytics, coding, UX/UI design and cybersecurity. Students in bootcamps gain real-world experience and quality instruction in a shorter amount of time. Upon completing a bootcamp, students can apply their new skills across industries — from retail companies to fintech.
Technology bootcamps typically offer:
- A comprehensive curriculum in a short time frame.
- Instruction methods and material for both experienced and inexperienced students.
- In-person and online options—and schedule flexibility for people who have other obligations, like a full-time job.
- An opportunity to build connections in the industry and develop the skills to excel in your career.
If you are interested in upskilling and gaining new skills within a few weeks or months, data science bootcamps are a readily available option. It is important to select a bootcamp that is offered through accredited universities and meet standards of quality, rigor, and reputation.
Short Courses
Online short courses provide individuals with an opportunity to complete non-credit bearing courses or earn a certificate. Short courses that allow you to earn a certificate typically offer sequential and progressive courses, similar to a degree program.
Data science short courses are offered in related subjects, including:
- Artificial intelligence (AI) and Machine Learning
- Cybersecurity
- Business analytics
- Data analytics
- Human-computer interaction
- Algorithmic trading
- FinTech
- Marketing Analytics
Individual self-paced courses vary in length but can take 48-90 hours to complete. Being able to work at your own pace is a perk for those who need greater flexibility or would like to learn faster or slower than the recommended cadence.
Even if you’re not looking to change careers, an online data science course can help expand your foundation of knowledge. Students who complete online data science courses gain valuable new skills to apply in their career.
CHOOSING THE BEST DATA SCIENCE MASTER’S PROGRAM FOR YOU
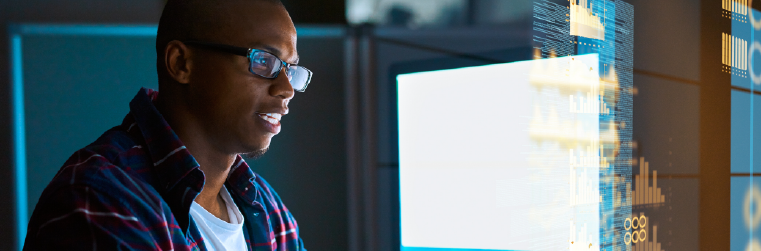
Online vs. On-Campus Data Science Programs
Both online and on-campus data science master’s programs can prepare students for careers in the field.
Traditional, campus-based learning offers:
- Face-to-face interaction with faculty and peers.
- Networking opportunities.
- Access to research materials and campus libraries among other facilities.
Online master’s programs tend to offer:
- Flexibility to complete coursework around work schedule and family life.
- Asynchronous course content, which means you can study on the fly from any location.
- Cost savings, since certain online programs may cost less than those offered in person.
Ready to take the next step to earn your master’s in data science?
Today, there are online data science degree programs designed to simulate an in-classroom experience and deliver a rigorous education to you in your home. When searching for the right online program for you, research the overall academic quality and credibility of the program to determine if it will suit your needs, learning style and career goals.
Full vs. Part-time Data Science Programs
Part-time data science degree programs typically appeal to students with limited time. Many universities accommodate a range of learner needs by offering both full- and part-time degree programs, in-person as well as online.
Full-time data science programs:
1-2 years to complete
Part-time data science programs:
2.5-3 years to complete
The only difference between these programs is that a part-time program may end up being double the length of a full-time program.
Determine how much time you have to commit to a program and how soon you wish to enter the field. If you are looking to become a data scientist sooner than later, pursuing a full-time program means you’ll earn your degree in a shorter amount of time. However, a part-time degree program in data science can open the door for the same types of career opportunities—with a little extra patience.
SPONSORED SCHOOLS

Syracuse University
School of Information Studies
Master of Science in Applied Data Science
Syracuse, New York
The online Master of Science in Applied Data Science at Syracuse University is an interdisciplinary program offered by the School of Information Studies and the Martin J. Whitman School of Management. The curriculum requires 36 credits, including electives that allow candidates to develop deep knowledge in their area of specialty. Students must compile a portfolio of projects to submit for faculty review. Online course content focuses on collaboration and includes face-to-face classes, project-based work, and 24/7 access to materials. Applicants must have a bachelor’s degree and must submit transcripts, a personal statement, resume, and two recommendations. No GRE Scores Required.
Enrollment Type: Full-Time and Part-Time
Length of Program: Complete in as few as 18 months
Credits: 34

The University of North Carolina at Chapel Hill
Master of Applied Data Science
Chapel Hill, NC
UNC-Chapel Hill’s online Master of Applied Data Science program gives you a holistic understanding of the data life cycle, preparing you to effectively — and ethically — collect, process, manage and analyze data. Learn to translate your insights into a clear narrative that can be used to drive action. Math and programming skills required.
Enrollment Type: Full-Time and Part-Time
Length of Program: As few as 16 months
Credits: 30

University of California, Berkeley
School of Information
Master of Information and Data Science
Berkeley, California
Designed for data science professionals, the UC Berkeley School of Information’s Master of Information and Data Science (MIDS) prepares students to derive insights from real-world data sets, using the latest tools and analytical methods to interpret and communicate their findings in ways that influence decision-making. The MIDS program features a rigorous, multidisciplinary curriculum focused on problem solving, preparing students to creatively apply methods of data collection, analysis, and presentation to address and solve problems in areas of human interest, government, and business.
Enrollment Type: Full-Time and Part-Time
Length of Program: As few as 12 months
Credits: 27
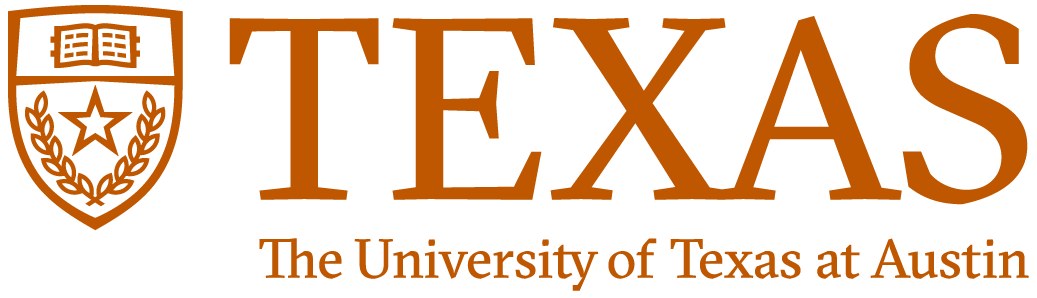
University of Texas at Austin
Master of Data Science
Austin, Texas
In today’s data-driven world, there’s an increased need for data scientists in every industry. Accelerate your career in this rapidly growing field by completing a rigorous master’s degree program that’s 100% online, affordable, and flexible to fit your life. With an MSDS from the top-ranked University of Texas at Austin, you’ll gain in-demand skills in data visualization, data mining, data analysis, machine learning, and more.
Enrollment Type: Full-Time and Part-Time
Length of Program: 1.5 – 3 Years
Credits: 30
Admission Requirements:
- Bachelor’s degree
- CV/Resume
- Statement of Purpose
- Transcripts from all universities attended
- Preparedness in Mathematics & Programming Preparation Form
- Quest Assessment
- TOEFL or IELTS – Required for International Students
- GRE scores – Currently Optional
- Letter(s) of recommendation (optional)
SPONSORED

Southern Methodist University
Dedman College of Humanities and Sciences, Lyle School of Engineering and Meadows School of the Arts
Master of Science in Data Science
Dallas, Texas
DataScience@SMU is an online Master of Science in Data Science program that prepares students with the fundamental skills needed to be sought-after data science leaders in a variety of industries. Our students learn from cross-disciplinary SMU faculty in online classrooms with a small student-to-professor ratio. DataScience@SMU students gain highly in-demand skills in working with unstructured data, big data processing, statistical analysis, text mining, machine learning, and business analytics.
Enrollment Type: Full-Time and Part-Time
Length of Program: Complete in as few as 20 months
Credits: 33.5